Seeking Transparency in Algorithmic Accountability with the Help of the SEC
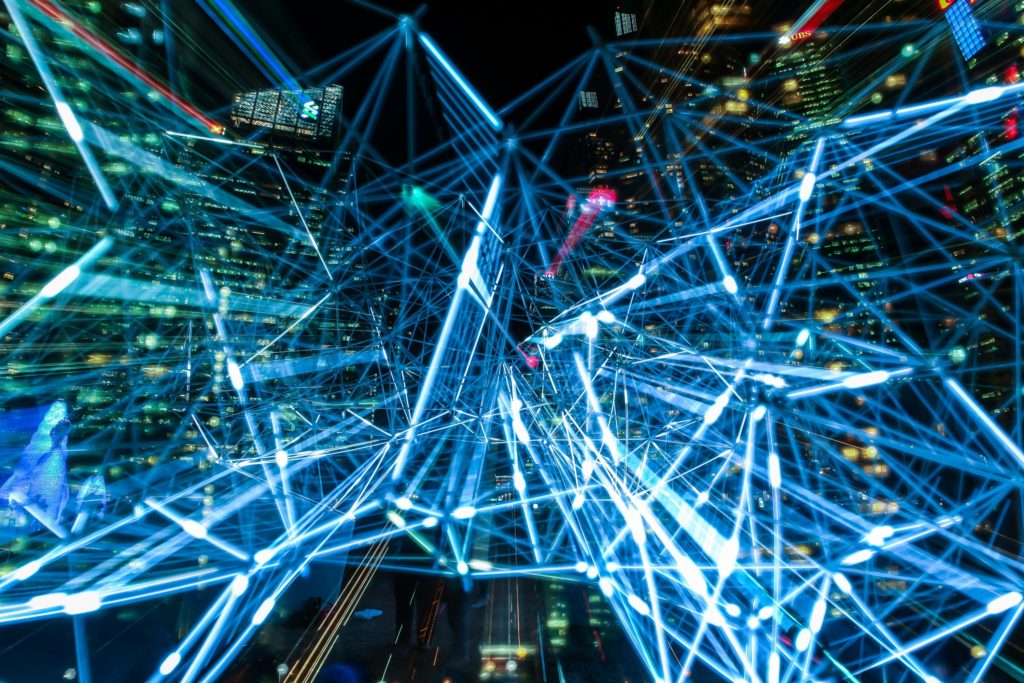
From the moment you wake up and check social media to the moment you end your day by streaming the latest binge-worthy TV show, chances are high that an algorithm is hard at work to help curate your “best life.” But the stakes are changing. Instead of simply recommending certain articles to read or movies to watch, algorithms are now increasingly being utilized to make much more impactful decisions in areas such as criminal justice or healthcare.
As a result, implementing a public policy that focuses on algorithmic accountability is becoming progressively more important. Ideally, such a policy would regulate the actions of both the private and public sectors with full and open transparency. By adopting a framework of public disclosure in a way that is similar to how the Securities and Exchange Commission currently regulates the financial markets, thoughtful leaders may be able to draft better legislation to achieve algorithmic accountability.
The 2019 Algorithmic Accountability Act (the Act) is the first national attempt at regulating algorithms, but as first attempts go, the Act produced more lessons learned and questions than actionable law. So far, critics have pointed out a need to reframe the issues as a means to better define what types of algorithms legislation should cover. For example, the size of a company may have little to do with the impact of an algorithm-assisted decision regarding the benefits of thousands, yet the size of a company is factored heavily in the Act. Others want to start from a broader perspective, suggesting legislators should begin by developing an algorithmic bill of rights. However, there is a serious issue with transparency in the Act that warrants at least as much attention as any other issue.
In the Act, the issue of transparency boils down to the optional nature of disclosing impact assessments of algorithms, or “automated decision systems,” to the public. For example, according to DataInnovation.org, impact assessments may help “evaluate how an automated system is designed and used—including the training data it relies on, the risks a system poses to privacy or security, and various other factors.” But ultimately, accountability takes a tumble because the Act allows impact assessments to be “made public by the covered entity at its sole discretion.” In other words, if a company deploys an automated decision system (ADS) that has serious privacy and security concerns, the public may never know about it. When considering that we live in a time where public scrutiny often tips the scales, the optional nature of disclosure hardly seems adequate.
To address issues of transparency, the Securities and Exchange Commission (SEC) may provide a model framework. In the U.S., the SEC is an agency that regulates how and what financial information companies must disclose to the public. However, the SEC does not determine whether any given company is good or bad. Instead, the responsibility of checking financial performance is ultimately shouldered by experts in the market. Similarly, for algorithms, regulators should consider enforcing a policy of mandatory disclosure while leveraging the power of the markets to collectively achieve accountability.
Public Sector Issues with Algorithms
Although both governments and companies have well-documented issues in deploying ADS, the 2019 Algorithmic Accountability Act curiously does not appear to cover government actions. However, if algorithms are deployed to help manage public benefits, omitting governments from regulatory oversight is a mistake that undercuts the premise of transparency.
The case study of Barry v. Lyon, 834 F.3d 706 (6th Cir. 2016), serves as just one example of why government actions must be regulated for algorithmic accountability. As profiled by the Litigating Algorithms 2019 US Report, the Michigan Department of Health and Human Services (MDHHS) deployed an algorithm to “automatically disqualify individuals from food assistance based on outstanding felony warrants.” However, the algorithm failed at technical, business, and legal levels.
The MDHHS algorithm demonstrated technical failure as it “improperly matched more than 19,000 Michigan residents, and automatically disqualified them from food assistance benefits with a vague notice.” Moreover, the algorithm failed a basic business logic test when Michigan projected it would cost $345,000 but produce “virtually no state savings.” From a legal perspective, courts eventually ruled that Michigan’s practices violated Federal statutes, the Supremacy Clause, and Constitutional due process requirements.
In the end, the state government paid for an algorithm that did not work and reversed decisions on benefits, while also paying out $3,120 to each class member who was unlawfully disqualified. Most importantly, real people suffered through years of lost benefits.
What if, instead, government agencies were subject to information disclosure requirements when deploying ADS in the same way that private sector companies must disclose their financial information to the SEC? Perhaps public scrutiny or the anticipation of such scrutiny could help other governments from becoming a MDHHS case study in the future.
Private Sector Issues with Algorithms
The government is not alone in failing to deploy an algorithm successfully. Private sector juggernauts, such as IBM and Microsoft, have also failed with their own ventures.
Joy Buolamwini’s research revealed that Microsoft and IBM released facial recognition algorithms that could detect the faces of men with light skin tones quite well but erred when detecting the faces of women with dark skin tones. In fact, in order to be recognized by cameras that used the facial recognition algorithms, Buolamwini had to put on a white mask. Aside from the obvious issues, a central problem with this case is that when Microsoft and IBM released these algorithms to the public without any disclosures, the algorithmic bias was unknowingly perpetuated.
How would things be different if Microsoft and IBM publicly disclosed benchmarks about their algorithms before external researchers exposed their biases? While it is difficult to speculate about the past, a public disclosure policy might have impacted Microsoft and IBM in a manner that is similar to how product liability currently works for consumer goods. Consider how one could apply product liability laws, which impose certain duties on producers, to the regulation of AI. For example, companies might have a duty to warn and subsequently a duty to test and disclose issues with their algorithms. Under this hypothetical framework, perhaps Microsoft and IBM could have avoided a public relations issue and released a better product. Further, researchers like Buolamwini, and camera manufacturers who implemented the algorithm in their products, would have had the opportunity to make a more informed decision about whether to use or improve the algorithms.
Conceivably, product-liability-inspired policy that follows the structure of the SEC disclosure regime could be the solution. Such public disclosure for tech companies is not new. Under the banner of protecting investors, companies like Microsoft and IBM submit financial disclosures to the SEC at least every quarter, and emerging companies go through extensive disclosure protocols when they go public in IPOs. Why can’t we protect consumers from harmful algorithms in the same way?
Regulating for Algorithmic Accountability
To regulate for algorithmic accountability, a future act should incorporate a policy of mandatory public disclosure in combination with the concept of product liability as a means to achieve algorithmic accountability. Such a policy would cover both public and private sector entities and require them to disclose how their algorithms are trained, what the intended uses are, and their associated performance benchmarks. Moreover, as algorithms learn and evolve from processing data, we should expect publicly available and understandable updates on how algorithms make or recommend decisions.
Challenges with a Disclosure Framework
Despite the potential advantages of pursuing a policy of public disclosure, there are a number of additional problems to consider. From the Great Depression to the Great Recession, information disclosure and regulation regimes have had a history of spectacular failures. However, we should see the following as a list of challenges to overcome rather than a list of excuses not to pursue the disclosure framework.
• Irrational Markets: People do not always behave rationally, and information disclosure may not prevent misuse—how do we manage bad actors and unintended outcomes?
• Privacy Concerns: Mandating information disclosure does not have to be mutually exclusive with privacy—how do we balance the two?
• Cost of Accountability: Registering securities in the United States is currently an expensive and resource-intensive endeavor—how can we make algorithmic registration an efficient process?
• Exemptions: Part of the complexity in securities regulation is understanding how to perfect an exemption—how can we determine what entities and what types of algorithmic decision-making systems to cover?
• Intellectual Property Rights: Companies like Coca-Cola have patents, trade secrets, copyrights, and trademarks, yet they still participate in the disclosure process for securities regulation—how can we protect intellectual property rights with a disclosure system?
• Product Liability: Laws governing product liability are existing examples of information disclosure that may apply to algorithmic accountability—how do efforts to regulate algorithms cross over?
Conclusion
Over the past decade, algorithms have permeated nearly every aspect of our lives. Since this article was first drafted in early 2020, the novel coronavirus (COVID-19) emerged to drastically change the world. Since then, COVID-19 has ushered in a new level of acceptance and even demand for more intrusive and sophisticated algorithms to help with tasks such as contact tracing. While there are presently more pressing issues concerning the larger economy and the health and wellness of frontline workers, there has also never been a more pressing need for algorithmic accountability. As legislators continue to explore different regulatory schemes, they should consider incorporating a policy of public information disclosure that promotes transparency as a pillar to ensure algorithmic accountability in both the public and private sectors.
Justin Chae is a Master of Science in Law student at Northwestern Pritzker School of Law.